Meilisearch MCP Server
A Model Context Protocol (MCP) server for interacting with Meilisearch through LLM interfaces like Claude.
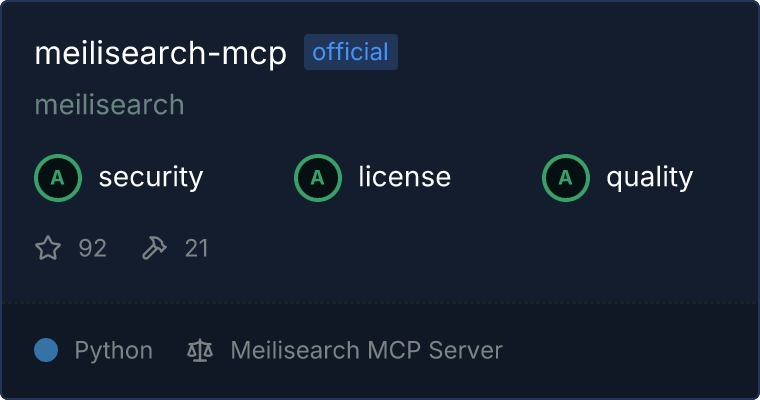
Features
Installation
# Clone repository
git clone <repository_url>
cd meilisearch-mcp
# Create virtual environment and install
uv venv
source .venv/bin/activate # On Windows: .venv\Scripts\activate
uv pip install -e .
Requirements
- Python ≥ 3.9
- Running Meilisearch instance
- Node.js (for testing with MCP Inspector)
Usage
Environment Variables
MEILI_HTTP_ADDR=http://localhost:7700 # Default Meilisearch URL
MEILI_MASTER_KEY=your_master_key # Optional: Default Meilisearch API key
Dynamic Connection Configuration
The server provides tools to view and update connection settings at runtime:
get-connection-settings
: View current connection URL and API key status
update-connection-settings
: Update URL and/or API key to connect to a different Meilisearch instance
Example usage through MCP:
// Get current settings
{
"name": "get-connection-settings"
}
// Update connection settings
{
"name": "update-connection-settings",
"arguments": {
"url": "http://new-host:7700",
"api_key": "new-api-key"
}
}
Search Functionality
The server provides a flexible search tool that can search across one or all indices:
search
: Search through Meilisearch indices with optional parameters
Example usage through MCP:
// Search in a specific index
{
"name": "search",
"arguments": {
"query": "search term",
"indexUid": "movies",
"limit": 10
}
}
// Search across all indices
{
"name": "search",
"arguments": {
"query": "search term",
"limit": 5,
"sort": ["releaseDate:desc"]
}
}
Available search parameters:
query
: The search query (required)
indexUid
: Specific index to search in (optional)
limit
: Maximum number of results per index (optional, default: 20)
offset
: Number of results to skip (optional, default: 0)
filter
: Filter expression (optional)
sort
: Sorting rules (optional)
Running the Server
python -m src.meilisearch_mcp
Usage with Claude Desktop
To use this with Claude Desktop, add the following to your claude_desktop_config.json
:
{
"mcpServers": {
"meilisearch": {
"command": "uvx",
"args": ["-n", "meilisearch-mcp"]
}
}
}
Testing with MCP Inspector
npx @modelcontextprotocol/inspector python -m src.meilisearch_mcp
Available Tools
Connection Management
get-connection-settings
: View current Meilisearch connection URL and API key status
update-connection-settings
: Update URL and/or API key to connect to a different instance
Index Management
create-index
: Create a new index with optional primary key
list-indexes
: List all available indexes
get-index-metrics
: Get detailed metrics for a specific index
Document Operations
get-documents
: Retrieve documents from an index with pagination
add-documents
: Add or update documents in an index
Search
search
: Flexible search across single or multiple indices with filtering and sorting options
Settings Management
get-settings
: View current settings for an index
update-settings
: Update index settings (ranking, faceting, etc.)
API Key Management
get-keys
: List all API keys
create-key
: Create new API key with specific permissions
delete-key
: Delete an existing API key
Task Management
get-task
: Get information about a specific task
get-tasks
: List tasks with optional filters:
limit
: Maximum number of tasks to return
from
: Number of tasks to skip
reverse
: Sort order of tasks
batchUids
: Filter by batch UIDs
uids
: Filter by task UIDs
canceledBy
: Filter by cancellation source
types
: Filter by task types
statuses
: Filter by task statuses
indexUids
: Filter by index UIDs
afterEnqueuedAt
/beforeEnqueuedAt
: Filter by enqueue time
afterStartedAt
/beforeStartedAt
: Filter by start time
afterFinishedAt
/beforeFinishedAt
: Filter by finish time
cancel-tasks
: Cancel pending or enqueued tasks
delete-tasks
: Delete completed tasks
System Monitoring
health-check
: Basic health check
get-health-status
: Comprehensive health status
get-version
: Get Meilisearch version information
get-stats
: Get database statistics
get-system-info
: Get system-level information
Contributing
- Fork repository
- Create feature branch
- Commit changes
- Create pull request
License
MIT